Article
When is a good time to introduce generative AI powered features in your product?
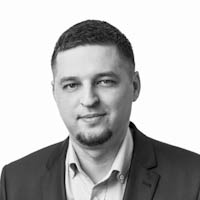
Maintaining a product roadmap is a difficult task, as there are many factors involved, with moving targets and continuously changing circumstances. This gets especially difficult when facing new technologies, which the team doesn’t have experience with. With generative AI models becoming more and more capable for everyday use we need to learn thinking with a completely new set of tools, unlocking potential ideas and solutions which we couldn’t even think about before. This needs extra creativity, but once the first ideas start popping up, come questions like: “Can we sell this feature?“, “Isn’t using generative AI too expensive?“, “Will people trust in such a feature?“ and of course: “Is this technology mature enough to include in our strategy?“
Exploring the readiness of AI for product integration
I could write, that more and more companies include generative AI wrapped some way in their product, but it could just be a phrase. Instead, let’s explore various aspects of the available offerings to judge how ready they are.
First, these AI capabilities are not just offered by startup companies. There are OpenAI models offered by Microsoft Azure, Gemini models offered by Google and also AWS has its Bedrock offering. Having such models offered by large organizations can guarantee, that the models will do their job as advertised and remain available. Capabilities improve at a rapid pace, so new and new versions of the models come out relatively frequently, so some maintenance effort should be considered, but in general the new services are backward compatible.
Next, if using models by trusted larger organizations we can be sure that our data is kept safe and not used for further training of the models, so our IP or other internal information will not be exposed or leaked to the public or competition.
Finally, the reliability of the generative AI outputs has improved over time dramatically. While hallucinations and wrong responses can still occur, such things can be partially managed by proper prompt engineering applied, and with proper UX we can present results in a way that even if there is some unexpected output, people will not lose trust in the product. The popularity of such services exploded in the past year because the benefits greatly outweigh the risks.
In short, if we watch out not to use the very first appearance of the new capabilities and use models by trusted organizations, we can safely say that if considering the limitations properly, these new generative AI features are indeed ready for production use.
Considerations for budgeting AI features in your product
The common perception is that running AI models, especially generative AI models, needs heavy resources and thus it’s expensive. But due to optimizations and the development in this field that is not necessarily the case anymore. Some smaller models can even run on a basic laptop, or on a PC with a basic gamer video card. LLMs (Large Language Models) are priced per token, where for simple calculations we can say that one word is one token, and with relatively good models (gpt-3.5-turbo for example) we can process 1 million tokens for just $1. Text processing is the cheapest option, some other services like multimodal GPT or image or video generation (for example $4 for 100 images) are of course somewhat more expensive.
The price of using generative AI is absolutely in the range where it is cheap enough to build features on top of, but if not used properly (for example exposing to the public in an unrestricted way), the costs can explode. When designing the new features, one needs to carefully consider the type of models to be used, the amount of prompting to be used (they can grow quite large in some cases) and how the new features are exposed to the end users. For some use cases they might only be used behind the scenes (like generating a product description from product details), which is predictable, in other cases there can be direct user interaction (like the AI helping the user creating a text). An experienced partner can help making informed and good decisions, keep the costs under control and remove risks and worry from the equation.
Crafting the right pricing and subscription structure
This leads to the need of coming up with a proper subscription model. Most companies offering an AI feature either make those features available for premium customers or as an extra separate subscription.
Once the cost of the usage has been estimated, a pricing model should be created that will cover the costs. There are typically two types of pricing models: monthly subscription or consumption based. Both have their benefits and downsides.
A subscription model is of course a good source of revenue - but only if people do subscribe. More and more AI features are offered in products for an extra subscription, and after the first few, people start hesitating to pay once more $20/month/user subscription… The problem with this model is that one needs to prepare for heavy users, who will use the AI features more, which brings the per-user cost up, but that may make the majority of users think that they need to pay more than the value offered. This could be helped by offering different tiers with different maximum usage of the AI related features.
On the other hand, consumption based models may make people worry about horrendous costs, if they some how can’t predict their usage. This can be alleviated by transparent pricing schemes (highlighting that average usage will not be expensive) and defining caps on the usage (relatively high upper limits, under fair use), preventing the explosion of costs.
In best case, if the usage costs of the AI features can be controlled (by limiting it through things like credits, or just predicting based on historical data), they could just be built into the overall pricing scheme as well. For any method you choose, the pricing needs to work based on some product related high level concept instead of tokens. End users will not want to bother with or even understand tokens. Instead, they need to know, how many articles they can get help with, how many itineraries they can make or if an unlimited usage is covered by their existing plan.
Transparency and responsible AI implementation
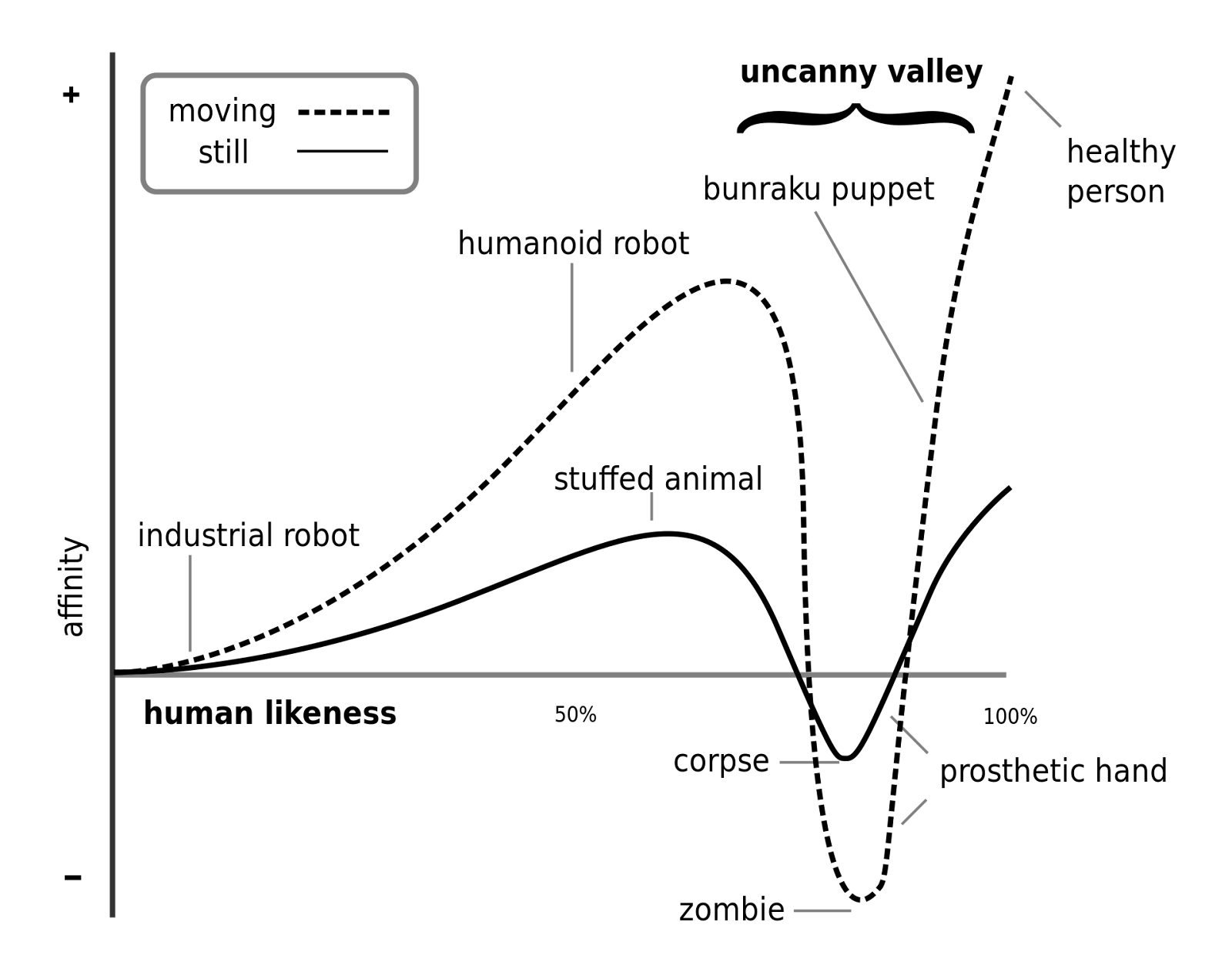
Finally, a concern that’s more difficult to address with typical economic logic is providing transparent and responsible AI features. Even a great idea can become a disaster if presented in a wrong way. What we need to avoid is the so called “Uncanny Valley”.
People don’t like to use automated features which are too simple, which just “don’t get it” - everyone dreads call center menu structures, where all they want is to connect to a human agent, but it’s impossible to find their way around. On the other hand, when something is presented as if it was coming from a human and it’s almost like human but not exactly (like an almost human-like robot which doesn’t blink), we feel unease and may become frustrated.
We shouldn’t present things in a form which they are not. In our experience AI supported features are accepted the most, when it is shown transparently what is coming from an AI. It could be a robot image, a cute name for the product’s “robot assistant” or other means.
Being responsible means that it’s not enough to design AI features and interactions well, but also be prepared for abuse and for incorrect output. Abuse can be prevented with proper prompts: we need to sanitize user inputs, similarly as avoiding SQL injections was a hot topic some years ago, but sanitation can also be done with LLM prompts. Some basic sanitation (content filtering) is also offered by the major model providers against hate, violence or sexual inputs. Incorrect output can be recognized with “grounding”, where generated output can be referenced with other materials to see if there is some hallucination ongoing.
The time is now: seizing the opportunities of generative AI in your product
The area of generative AI is rapidly moving and growing, and their offering is now mature enough to be wrapped and included in both B2B and B2C products. The market is slowly awakening and just starting to realize the potential in this next revolution of information technology. As generative AI will be a more common tool in our toolset and using it becoming the norm, people will get more and more ideas on how to utilize in their products.
One can get ahead of the competition by seizing the moment and with conscious planning and strategy. Educate yourself on what generative AI can do for you, expand the horizon of ideation, plan it into your roadmap, and with taking the above pieces of advice seriously, you have all the ingredients to drive your product to success.
New and new capabilities will continue to be released, so it’s best to keep having an open mind about the new features and which of them become stable and mature enough. In the past year, besides simply using raw GPT prompts we now have function calling, RAG, multimodal models (to say just a few buzzwords), and OpenAI just recently announced the “4o” model, which will be able to communicate via voice almost in real time. Who knows, in what ways we will further improve our products next year…